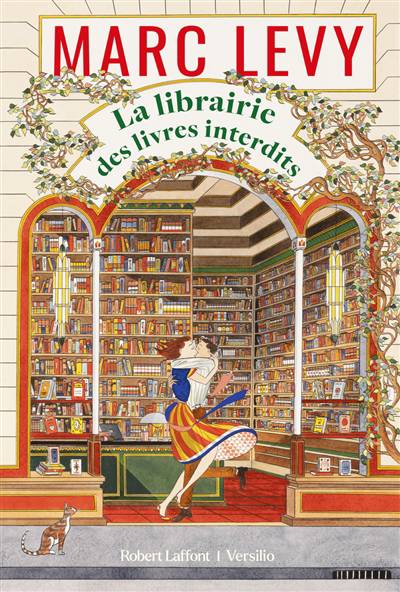
This book presents the major advances in techniques to preserve privacy and security requirements connected with the use of AI and machine learning (ML) to analyse and manage sensitive data in MCPSs. The advances in Internet of things and artificial intelligence (AI) have witnessed great progress on healthcare technologies in several application domains. In particular, the interconnection between the physical spaces, characterized by physical devices able to collect users' health information, with the cyberspace, also known as the virtual space, has fostered the development of intelligent Medical Cyber-Physical Systems (MCPSs) with the capability to deliver real-time healthcare services. On the other hand, the potential innovation that these technologies bring to improve patient care, by remotely analysing health parameters using medical devices, advanced smart sensors, and AI, is hampered by security and privacy challenges related to the managed sensitive data.
Starting from the state of the art on AI and ML for medical applications and digital health, an accurate analysis of privacy and security risks associated with the use of the MCPSs is presented. Then, Digital Twins are introduced as a significant technique to enhance decision-making through learning and reasoning of collected on-field real-time data. Moreover, decentralized healthcare data management approaches based on federated learning, tiny machine learning, and blockchain technologies have been introduced to shift control and responsibility of healthcare data management from individual centralized entities to a more distributed structure, preserving privacy and security. Finally, the application of AI-based security monitoring approaches in healthcare is discussed.
In this book, both theoretical and practical approaches are used to allow readers to understand complex topics and concepts easily also through real-life scenarios.