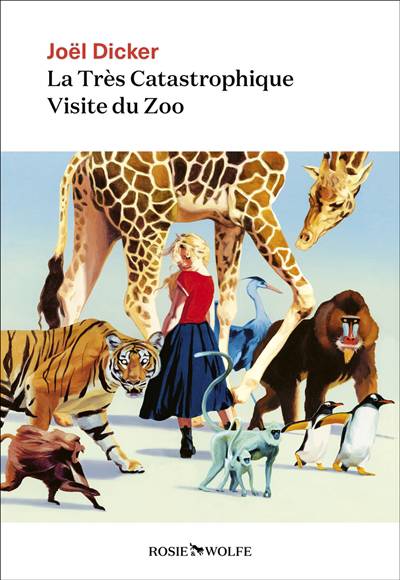
In recent years, embodied multi-agent systems, including multi-robots, have emerged as essential solution for demanding tasks such as search and rescue, environmental monitoring, and space exploration. Effective collaboration among these agents is crucial but presents significant challenges due to differences in morphology and capabilities, especially in heterogenous systems. While existing books address collaboration control, perception, and learning, there is a gap in focusing on active perception and interactive learning for embodied multi-agent systems.
This book aims to bridge this gap by establishing a unified framework for perception and learning in embodied multi-agent systems. It presents and discusses the perception-action-learning loop, offering systematic solutions for various types of agents homogeneous, heterogeneous, and ad hoc. Beyond the popular reinforcement learning techniques, the book provides insights into using fundamental models to tackle complex collaboration problems.
By interchangeably utilizing constrained optimization, reinforcement learning, and fundamental models, this book offers a comprehensive toolkit for solving different types of embodied multi-agent problems. Readers will gain an understanding of the advantages and disadvantages of each method for various tasks. This book will be particularly valuable to graduate students and professional researchers in robotics and machine learning. It provides a robust learning framework for addressing practical challenges in embodied multi-agent systems and demonstrates the promising potential of fundamental models for scenario generation, policy learning, and planning in complex collaboration problems.
Nous publions uniquement les avis qui respectent les conditions requises. Consultez nos conditions pour les avis.