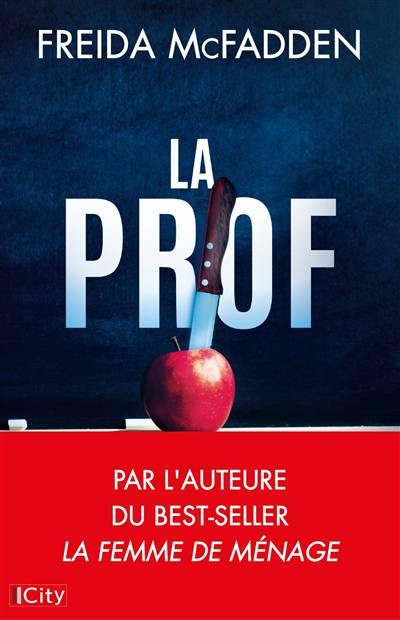
This volume presents a different, yet logically unassailable, view of statistical modeling. It details a method of modeling based on the principle that the objective is to extract the information from data that can be learned with suggested classes of probability models. The intuitive and fundamental concepts of complexity, learnable information, and noise are formalized, which provides a firm information theoretic foundation for statistical modeling. The view of the modeling problem presented in this book permits a unified treatment of any type of parameters, their number, and even their structure. Since only optimally distinguished models are worthy of testing, a logically sound and straightforward treatment of hypothesis testing is found in which the confidence in the test result can be assessed. The techniques presented in this book have application in all fields of modern engineering, including signal and image processing, bioinformatics, pattern recognition, and machine learning.
Nous publions uniquement les avis qui respectent les conditions requises. Consultez nos conditions pour les avis.