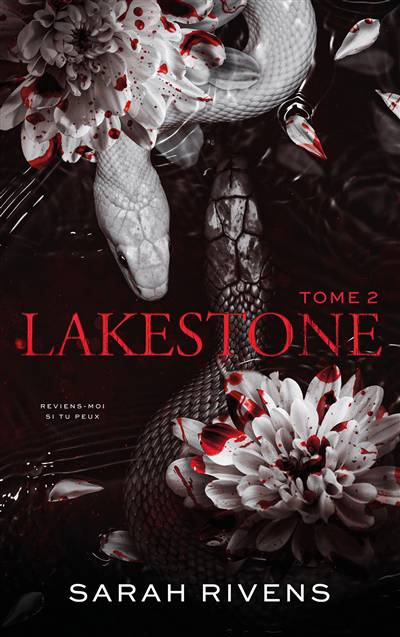
In this book we will develop Machine Learning techniques related to non-linear regression. More specifically, we will go deeper into non-linear multiple regression models with all their identification, estimation and diagnosis problems. Special emphasis is placed on generalised linear models and all types of derived non-linear models: Logit Models, Probit Models, Poisson Models and Negative Binomial Models. This is followed by models of limited dependent variable, discrete choice, count, censored, truncated and sample selection. Non-linear models with panel data are also discussed in depth. An important section is devoted to predictive models of neuroanalytic networks. All chapters are illustrated with examples and representative exercises solved with the latest software such as R, SAS, SPSS, EVIEWS and STATGRAPHICS.
Nous publions uniquement les avis qui respectent les conditions requises. Consultez nos conditions pour les avis.