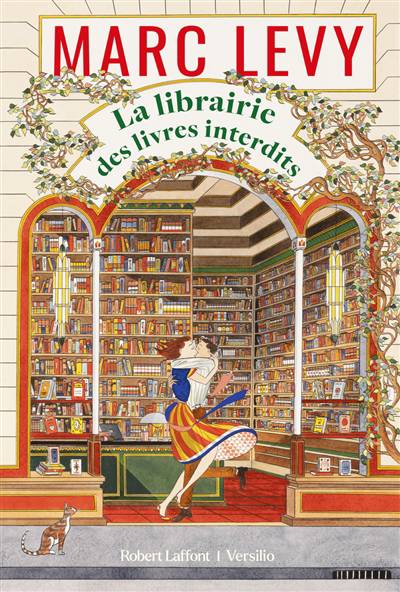
"Mathematics for Machine Learning: A Deep Dive into Algorithms" is a comprehensive guide that bridges the gap between mathematical theory and practical applications in the dynamic world of machine learning. Whether you're a data science enthusiast, a budding machine learning engineer, or a seasoned practitioner, this book equips you with the essential mathematical foundations that power cutting-edge algorithms and data-driven insights.
Starting with the fundamentals of linear algebra, multivariable calculus, probability, and statistics, Nibedita expertly guides you through the intricate maze of mathematical concepts. From there, you'll explore the depths of linear regression, classification, support vector machines, neural networks, and more, all while unraveling the underlying mathematical principles that make these algorithms tick.
This book isn't just about equations and formulas—it's about unlocking the potential of machine learning through a strong mathematical intuition. Nibedita's clear explanations, illustrative examples, and practical insights ensure that you not only grasp the core concepts but also discover how they translate into real-world solutions. Dive into the intricacies of convolutional and recurrent neural networks, grasp the significance of regularization techniques, and explore the ethical dimensions of AI and machine learning.
Whether you're seeking to build a solid foundation for a career in data science or aiming to deepen your understanding of machine learning algorithms, "Mathematics for Machine Learning" empowers you to harness the power of mathematics as a tool for innovation and transformation in the digital age.