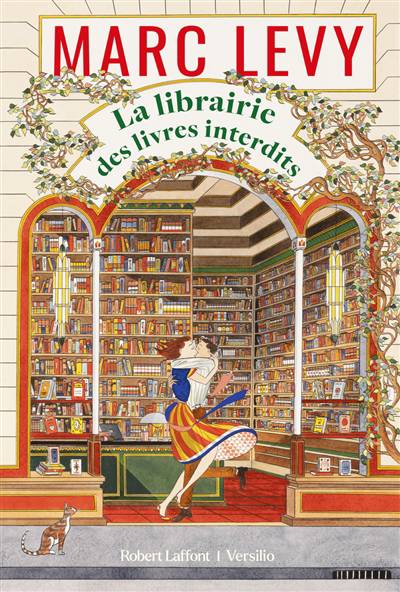
Shimon Edelman bases a comprehensive approach to visual representation on the notion of correspondence between proximal (internal) and distal similarities in objects.
Researchers have long sought to understand what the brain does when we see an object, what two people have in common when they see the same object, and what a seeing machine would need to have in common with a human visual system. Recent neurobiological and computational advances in the study of vision have now brought us close to answering these and other questions about representation.
In Representation and Recognition in Vision, Shimon Edelman bases a comprehensive approach to visual representation on the notion of correspondence between proximal (internal) and distal similarities in objects. This leads to a computationally feasible and formally veridical representation of distal objects that addresses the needs of shape categorization and can be used to derive models of perceived similarity.
Edelman first discusses the representational needs of various visual recognition tasks, and surveys current theories of representation in this context. He then develops a theory of representation that is related to Shepard's notion of second-order isomorphism between representations and their targets. Edelman goes beyond Shepard by specifying the conditions under which the representations can be made formally veridical. Edelman assesses his theory's performance in identification and categorization of 3D shapes and examines it in light of psychological and neurobiological data concerning the object-processing stream in primate vision. He also discusses the connections between his theory and other efforts to understand representation in the brain.