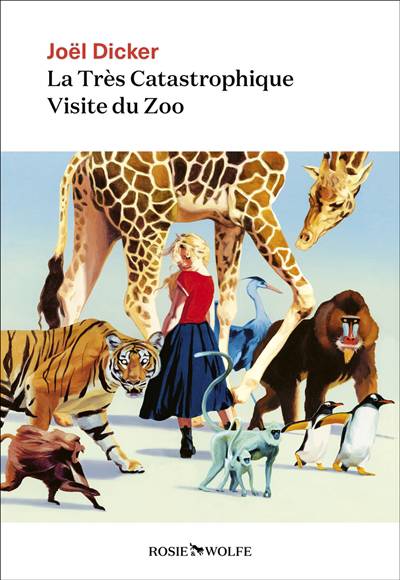
This book aims to provide a comprehensive understanding of tensor computation and its applications in seismic data analysis, exclusively catering to seasoned researchers, graduate students, and industrial engineers alike. Tensor emerges as a natural representation of multi-dimensional modern seismic data, and tensor computation can help prevent possible harm to the multi-dimensional geological structure of the subsurface that occurred in classical seismic data analysis.
It delivers a wealth of theoretical, computational, technical, and experimental details, presenting an engineer's perspective on tensor computation and an extensive investigation of tensor-based seismic data analysis techniques. Embark on a transformative exploration of seismic data processing-unlock the potential of tensor computation and reshape your approach to high-dimensional geological structures.
The discussion begins with foundational chapters, providing a solid background in both seismic data processing and tensor computation. The heart of the book lies in its seven chapters on tensor-based seismic data analysis methods. From structured low-tubal-rank tensor completion to cutting-edge techniques like tensor deep learning and tensor convolutional neural networks, each method is meticulously detailed. The superiority of tensor-based data analysis methods over traditional matrix-based data analysis approaches is substantiated through synthetic and real field examples, showcasing their prowess in handling high-dimensional modern seismic data. Notable chapters delve into seismic noise suppression, seismic data interpolation, and seismic data super-resolution using advanced tensor models. The final chapter provides a cohesive summary of the conclusion and future research directions, ensuring readers facilitate a thorough understanding of tensor computation applications in seismic data processing. The appendix includes a hatful of information on existing tensor computation software, enhancing the book's practical utility.
Nous publions uniquement les avis qui respectent les conditions requises. Consultez nos conditions pour les avis.