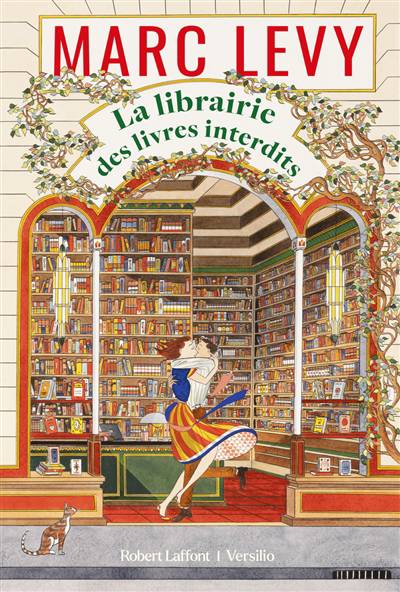
This book investigates in detail the emerging deep learning (DL) technique in computational physics, assessing its promising potential to substitute conventional numerical solvers for calculating the fields in real-time. After good training, the proposed architecture can resolve both the forward computing and the inverse retrieve problems.